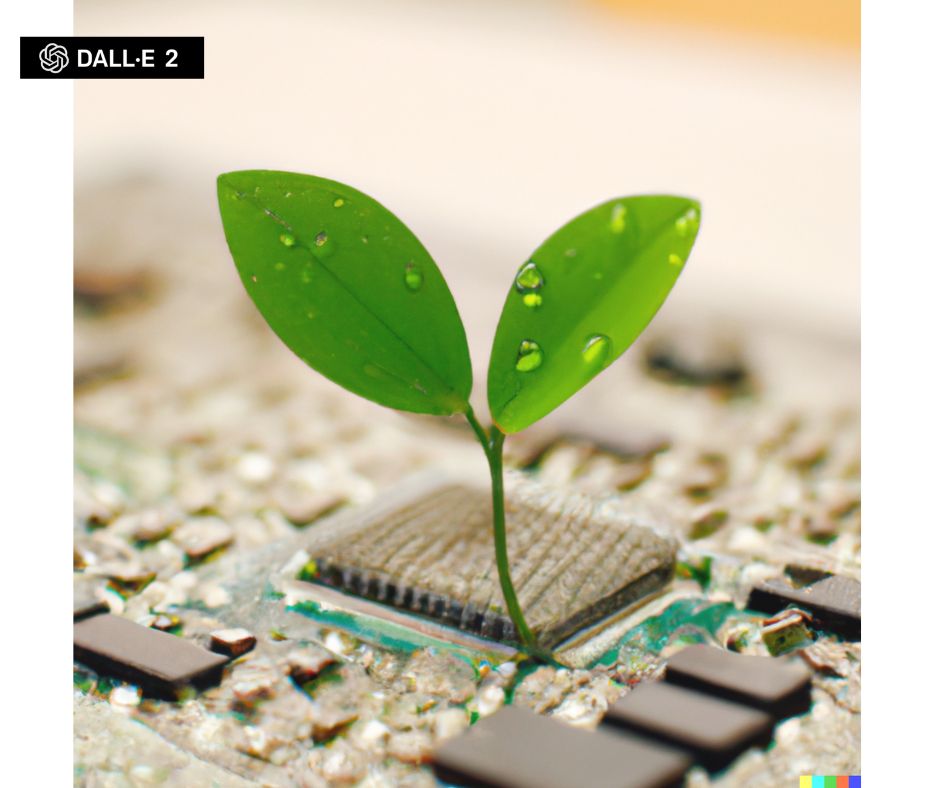
Can AI reverse climate change?
It’s hard to find a topic more present in everyday discussions than AI at the moment. With the success of OpenAI’s CHatGPT, everyone has suddenly realized the potential that machine learning, natural language processing, and neural networks have – and we’re probably still scratching the surface.
But besides potentially taking over the world, building an endless amount of paperclips, and making lots of jobs redundant, are there areas within sustainability where AI can help us transition more quickly to a 1.5-degree world, avoiding the worst impacts of climate change? How can AI be used to reverse climate change? And finally, what effect does AI have on the climate?
Energy systems
- Forecasting electricity supply and demand: To plan and schedule electricity effectively, it is necessary to anticipate both the supply and demand of power in advance. Machine learning (ML) can enhance these predictions by increasing their accuracy, enhancing temporal and spatial resolution, and measuring the degree of uncertainty. (Check out: https://www.myst.ai/services/)
- Renewable Energy: One of AI’s most potential applications in this field is in renewable energy. By utilizing machine learning algorithms, energy companies can now predict the output of sources such as wind and solar power with significantly improved accuracy, resulting in more efficient and sustainable use of energy. This, in turn, can contribute to reducing greenhouse gas emissions, improving energy security, and decreasing the expenses related to renewable energy production. (Check out: https://www.tomorrow.io/)
- Improving scheduling and flexible demand: Power grid scheduling algorithms face difficulties in managing sizeable amounts of time-varying electricity sources such as solar and wind power. However, machine learning (ML) can enhance these algorithms, enabling better management of storage and flexible demand, designing real-time electricity prices that minimize CO2 emissions. (Check out: https://www.auto-grid.com/)
- Nuclear Fusion: Although nuclear fusion has the potential to generate electricity that is both safe and carbon-free, the reactors currently consume more energy than they produce. Machine learning (ML) can contribute by proposing parameters for physical experiments and modeling plasma behavior within reactors. While extensive scientific and engineering research is still necessary, ML can accelerate the process by providing guidance for experimental design and monitoring physical processes. Fusion reactors require smart experimental design because of the large number of adjustable parameters, and ML can assist in prioritizing which parameter settings should be explored during physical experiments. As an example, Google and TAE Technologies have collaborated on a human-in-the-loop experimental design algorithm that enables TAE’s reactor to perform rapid parameter exploration. (Check out: https://www.magics.tech/about/)
- Reducing life cycle fossil fuel emissions: Apart from the inescapable environmental effects of using fossil fuels, natural gas pipelines tend to release methane, a potent greenhouse gas. Machine learning (ML) has the potential to identify and avoid such leaks until society can transition away from natural gas usage. (Check out: https://kairosaerospace.com/)
Mobility
- Electric vehicles: Machine learning (ML) is crucial for addressing various issues associated with electric vehicles (EVs). ML can enhance charge scheduling, congestion management, and vehicle-to-grid algorithms. Furthermore, ML techniques have been employed to improve battery energy management, such as estimating or optimizing charging in hybrid EVs and detecting faults and lateral misalignment in wireless charging of EVs. With the availability of in-vehicle sensors and communication data, there is an opportunity to comprehend the travel and charging behavior of EV owners, which can guide the placement of charging stations. Since battery electric vehicles are mostly idle for much of the day, they can serve as energy storage for the grid at other times, with charging and discharging controlled by price signals. There is significant potential for ML to enhance vehicle-to-grid technology using methods like reinforcement learning, which can reduce greenhouse gas emissions resulting from electricity generation. (Check out: https://met3r.com/)
- Freight routing and consolidation: Efficiently directing and merging freight shipments can significantly diminish emissions. Machine learning (ML) can assist in optimizing freight transportation by collecting data on suppliers, freight demand, and transit delays and utilizing this information to produce optimal transportation schedules. (Check out: https://www.optiyol.com/)
- Self-driving cars: Autonomous vehicles have the potential to transform transportation by decreasing the number of cars on the road and the amount of fuel consumed. Machine learning plays a crucial role in the development of self-driving cars, such as performing fundamental tasks like tracking the road and identifying obstacles. While autonomous cars can potentially decrease energy consumption by alleviating traffic congestion and implementing eco-driving methods, it is also feasible that they might prompt a surge in overall road traffic, which can negate efficiency benefits. (Check out: https://www.Tesla.com)
Buildings
- Modeling building energy: To enhance the energy efficiency of buildings, it is crucial to comprehend the growing volume of data generated by meters and energy monitors in homes. Machine learning (ML) can be utilized to simulate the energy needs of buildings, quantify the energy consumption of specific appliances, and assess the efficiency of energy-saving measures. (Check out: https://new.abb.com/buildings/smarter-building)
- Smart Appliances: Intelligent appliances and control systems can aid buildings in reducing their carbon emissions. By utilizing machine learning (ML), heating and cooling systems can be managed more effectively, lighting and temperature can be adjusted according to occupancy patterns, and energy consumption can be altered in response to energy prices that are sensitive to carbon emissions. (Check out: https://www.hank.re/)
Farms and Forests
- Precision agriculture: Conventional industrial agriculture practices contribute to the release of CO2 into the atmosphere by disturbing the natural chemistry and diversity of the soil. Furthermore, the use of chemicals required in this type of agriculture is emissions-intensive, both during their production and application. Machine learning (ML) can aid in monitoring emissions, reducing the dependence on chemicals by identifying pests, diseases, and weeds, and revolutionizing agricultural practices by utilizing physical robots. (Check out: https://agro-scout.com/)
- Modelling carbon stock: Modeling (and pricing) carbon stored in forests requires us to assess how much is being sequestered or released across the planet. ML can help identify tree species and heights from satellite and aerial imagery. (Check out: https://pachama.com/)
- Afforestation: Strategic tree planting in suitable areas can assist in carbon sequestration and potentially enhance local ecosystems, provided it is done cautiously. Machine learning (ML) can assist in identifying appropriate locations for tree planting, monitoring the health of the plants, identifying and managing weeds, analyzing patterns and tendencies, and even utilizing drone technology to plant trees. (Check out: https://dendra.io/)
Physical systems
- Climate systems: Accurate measurements of environmental emissions play a crucial role in developing models that can simulate climate scenarios in the future. Artificial intelligence (AI) can assist in this process by providing more precise measurements and by processing remote-sensing data from satellites. Additionally, AI can be utilized to monitor the effectiveness of climate policies, which can help teams improve their climate models and strategies for mitigating climate change. (Check out: https://climatetrace.org/our-approach)
- Risk analysis and environment: Artificial Intelligence (AI) is utilized to observe and record shifts in the environment, enabling prediction and response to environmental crises such as wildfires, droughts, and hurricanes. Machine learning algorithms can be merged with satellite imagery and drone technology to track and oversee alterations in land usage, water supply, and weather trends, supplying vital data to decision-makers to aid in the reduction of climate change impacts. (Check out: https://cervest.earth/)
The carbon footprint of AI
Ok, the consensus amongst climate and earth scientists is that machine learning models are powerful tools. Used wisely, machine learning has the potential to make climate science more widely available and applicable through the industrialized analysis of data. But what effect does it itself have on emissions, what’s its carbon footprint?
To evaluate the carbon footprint of a machine learning model, it is possible to differentiate three types:
- a) The carbon footprint generated during the training of the model,
- b) The carbon footprint produced when the model is used for inference after it has been deployed,
- c) The overall carbon footprint of the model throughout its lifespan.
To calculate the carbon footprint of any model, regardless of its type, two key pieces of information are necessary:
- the quantity of electricity it uses and
- the carbon intensity of that electricity.
# 1 depends a lot on the hardware it’s running on, as well as the utilization rate of the hardware.
# 2 depends a lot on how the electricity is produced, for instance, solar energy and wind energy are obviously much greener than coal for instance. To quantify this, one often uses the average carbon intensity of electricity in the grid in which the hardware is located.
The artificial-intelligence industry is often compared to the oil industry: once mined and refined, data, like oil, can be a highly lucrative commodity. Now it seems the metaphor may extend even further. Like its fossil-fuel counterpart, the process of deep learning has an outsize environmental impact.”
-Karen Hao, MIT Technology Review (Karen Hao, 2019)
MIT reported that the cloud now has a larger carbon footprint than the entire airline industry, and a single data center might consume an amount of electricity equivalent to 50,000 homes. In a new paper, researchers at the University of Massachusetts, Amherst, performed a life cycle assessment for training several common large AI models. They found that the process can emit ca. 300 tons of carbon dioxide equivalent—nearly five times the lifetime emissions of the average American car (and that includes the manufacture of the car itself).
Want to estimate the footprint of your AI model?
- The Machine Learning Emissions Calculator https://mlco2.github.io/impact/#compute can help practitioners run estimations based on factors like cloud provider, geographic region, and hardware.
- Best practices to reduce the footprint of your AI model: https://ai.googleblog.com/2022/02/good-news-about-carbon-footprint-of.html
- And if you don’t care about sustainability but instead want to see how you can build as many paperclips as possible, we really enjoyed this game. (the beginning is slow, but it takes off pretty quickly) https://www.decisionproblem.com/paperclips/
Other noteworthy links:
ChatIPCC can converse about climate science, using the latest and most authoritative scientific papers as its data input.
Do you want to know how we can help your company become more sustainable?
Book a short intro call with our Head of Climate Philip Reuchlin who will introduce you to the Pioneers offerings. Just send a quick mail to philip.reuchlin@pioneers.io.
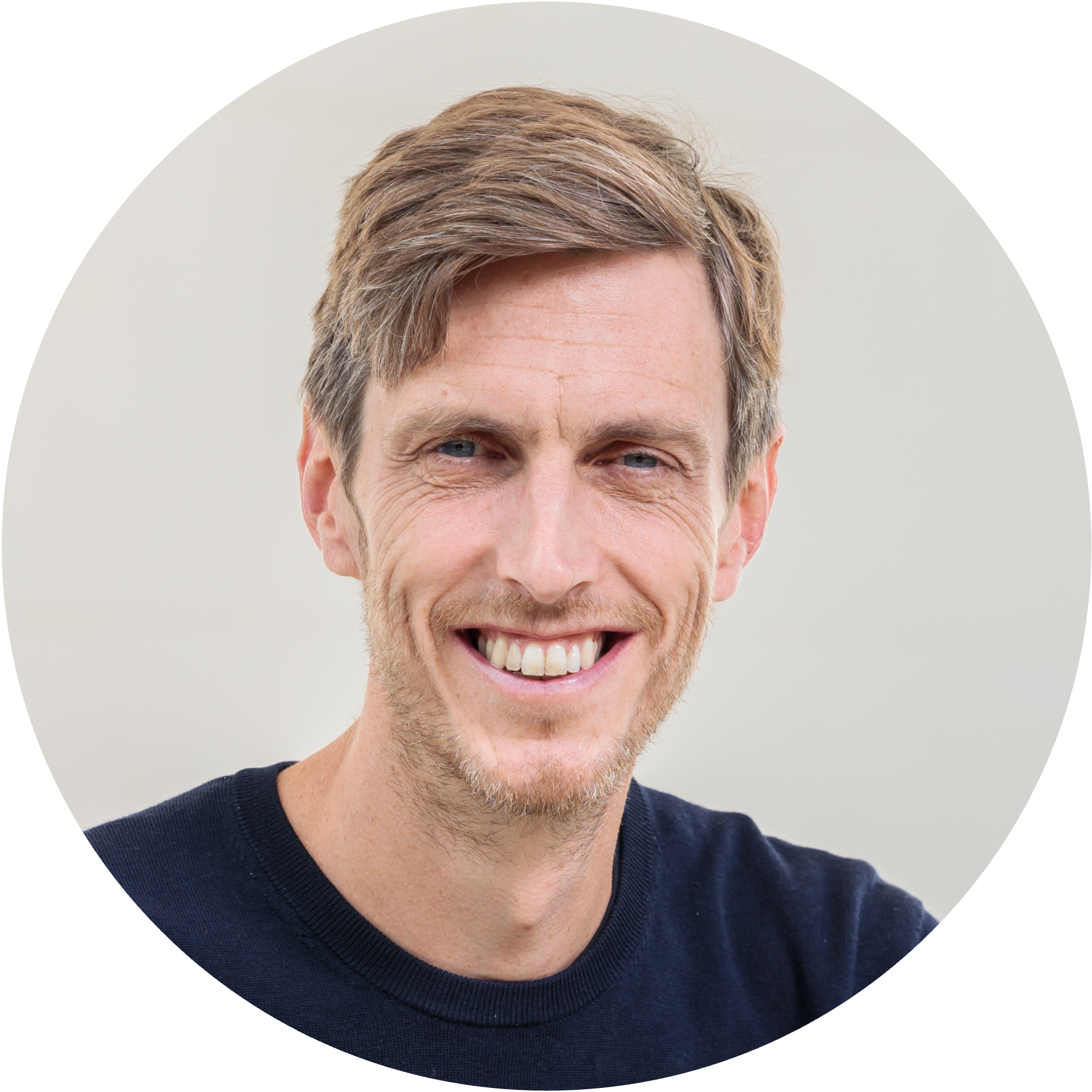
Philip Reuchlin
Head of Climate